The Root of AI Bias in Health Insurance – Insufficiently Diverse Input Data
AI is now becoming mainstream. You’re starting to see them integrated into as many workflows as possible. AI has really taken off in the administrative space and is going to continue to do so.
Among the industries that AI is taking over, lots of people are talking about artificial intelligence (AI) in healthcare these days, particularly algorithms that can identify individuals at risk for lung cancer. The hope is that AI will soon provide real-time healthcare recommendations. However, there is growing excitement that these tools can improve healthcare, but there is also a risk that they will perpetuate long-standing inequities.
Before getting into those risks, let’s look at what AI can do to help healthcare, especially health insurance better.
How Can AI Take on the Pain Point of Health Insurance System?
The health insurance industry is facing many challenges such as privacy, increasing competition, rising costs, and an aging population. Managing prescription coverage benefits can be challenging today, especially if patient care involves a complicated illness or injury and multiple types of medication.
Healthcare fraud costs the US about $300 billion annually, with health insurance fraud roughly accounting for $100 billion. This fraud burden results in higher premiums for customers. AI models can effectively detect and prevent fraud by automating manual workflows. Insurers traditionally review claims manually, a time-consuming and inefficient process.
AI, particularly NLP-based models, can reduce the time required to identify suspicious claims by 75%, thus lowering the rate of reinvestigated claims from an unrealistic 70%.
According to Deloitte, up to 75% of customer service requests can be automated using chatbots, leading to a 40% reduction in customer service costs and freeing employees’ time by up to 97%. AI-driven chatbots, specifically trained for health insurance needs, can handle simple queries, extract relevant data to establish policies, and manage insurance claims.
In 2019, private health insurance spending in the US was nearly $1.2 trillion, accounting for about 30% of total healthcare spending. Companies are seeking a competitive edge by implementing AI in this vast market. Many tasks in health insurance practices are traditionally done manually, but 72% of health insurance executives consider investing in AI a top strategic priority for 2022.
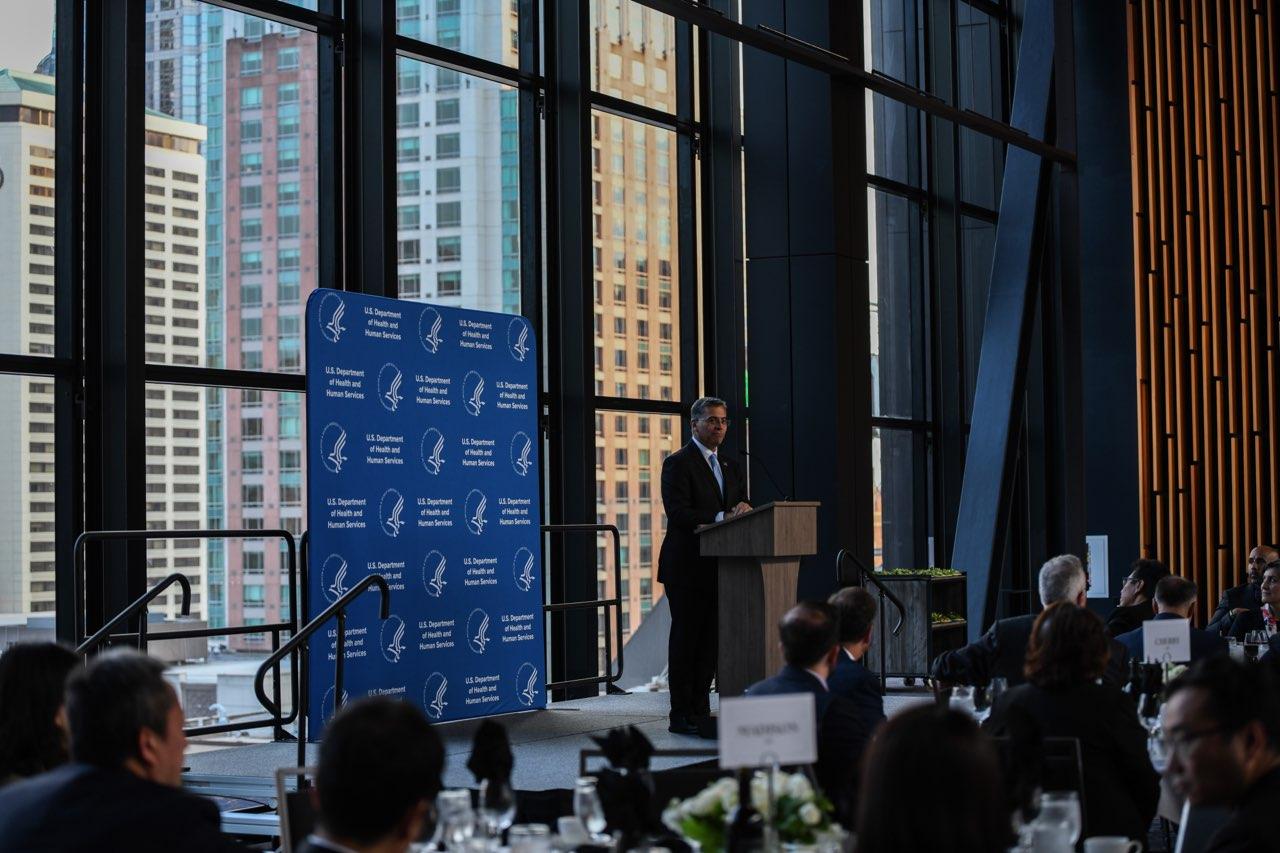
Health insurance companies are encouraging healthier lifestyles by lowering premiums. For instance, John Hancock introduced an interactive policy in 2018 that collects health data via smartphones and wearables. This integration of AI and IoT allows health insurers to conduct an effective and fair underwriting process based on a customer’s life choices.
As the industry began exploring positive changes in customer healthcare with AI innovations, the prescription coverage benefit highlights the advantage of AI in consumer choices for health insurance. Each health insurance carrier approaches coverage and pricing for medication differently. AI can assist in the purchase of health insurance and guide an applicant to the insurance company that best matches their prescription needs, including affordability.
Although AI does confer significant benefits to the health insurance industry, there are significant risks and challenges that need to be overcome that can slow down the rate of adoption or, in some cases, prevent the adoption of AI-based solutions. Recently, many studies have shown that adopting AI that’s trained on insufficiently diverse data can lead to AI bias.
AI Bias in Health Insurance and Its Roots
There is growing concern that algorithms may reproduce racial and gender disparities via the people building them or through the data used to train them. Empirical work is increasingly lending support to these concerns.
For example, job search ads for highly paid positions are less likely to be presented to women, searches for distinctively Black-sounding names are more likely to trigger ads for arrest records, and image searches for professions such as CEO produce fewer images of women. Facial recognition systems increasingly used in law enforcement perform worse on recognizing faces of women and Black individuals, and natural language processing algorithms encode language in gendered ways.
What Did Researchers Say?
Health systems rely on commercial prediction algorithms to identify and help patients with complex health needs. In a study “Dissecting racial bias in an algorithm used to manage the health of populations” published on Science, researchers show that a widely used algorithm, typical of this industry-wide approach and affecting millions of patients, exhibits significant racial bias.
According to the study, at a given risk score, Black patients are considerably sicker than White patients, as evidenced by signs of uncontrolled illnesses. Remedying this disparity would increase the percentage of Black patients receiving additional help from 17.7 to 46.5%. The bias arises because the algorithm predicts health care costs rather than illness, but unequal access to care means that we spend less money caring for Black patients than for White patients.
A 2019 study by Obermeyer et al. revealed that a widely used health algorithm assigned Black patients the same health risk scores as white patients, even though the Black patients were sicker. This occurred because the algorithm used healthcare costs as a proxy for health, reflecting the inequitable allocation of resources rather than actual health status.
A 2022 study by Gichoya et al. found that deep learning models could identify a patient’s race from medical images with no explicit racial markers, a task impossible for human experts. This ability poses a risk of unintended racial recognition by AI models, which can exacerbate biases if not properly managed.
The Roots of AI Bias – Insufficiently Trained on Non-Diverse Data
This bias stems from AI models trained on non-diverse datasets, mirroring the historical reliance on predominantly white patient data in medical research. Such biases can result in significant disparities in healthcare quality and outcomes for minority patients.
Edward Lee, MD, executive vice president and chief information officer of The Permanente Federation, an important figure in the healthcare community, emphasizes that it is crucial for the healthcare industry to recognize that AI algorithms trained on insufficiently diverse data can lead to AI bias.
This bias can inadvertently contribute to widening healthcare disparities. One of the first steps to combat this issue is to be intentional in looking for bias; if we don’t look, we’ll never find it. Understanding that AI bias can be part of any algorithm is essential. Ultimately, Lee considers AI to be augmented intelligence, not simply artificial intelligence. It is most impactful when used as a tool to augment, assist, and complement physicians’ clinical decision-making rather than as standalone technology.
Government Efforts to Minimize the Risks of AI Bias
More states in the U.S. are seeking to regulate (or at least monitor) its use. Many are passing legislation, issuing policy rules or forming committees to inform those decisions.
The regulation of health insurers in the U.S. varies based on the type of health insurance. For Medicaid, each state and U.S. territory operates its program within federal guidelines set by the Centers for Medicare and Medicaid Services (CMS). CMS provides summaries and oversight of these programs and has recently issued rules regarding the use of AI in prior authorization processes, emphasizing innovation, security, and the reduction of administrative burdens.
In February 2024, CMS also addressed AI use in Medicare Advantage plans, requiring insurers to ensure AI-assisted coverage decisions comply with anti-discrimination rules. Commercial health plans, covering about two-thirds of Americans, are regulated by individual states. Many states are introducing or passing legislation to regulate AI in healthcare, influenced by guidelines from the National Association of Insurance Commissioners (NAIC).
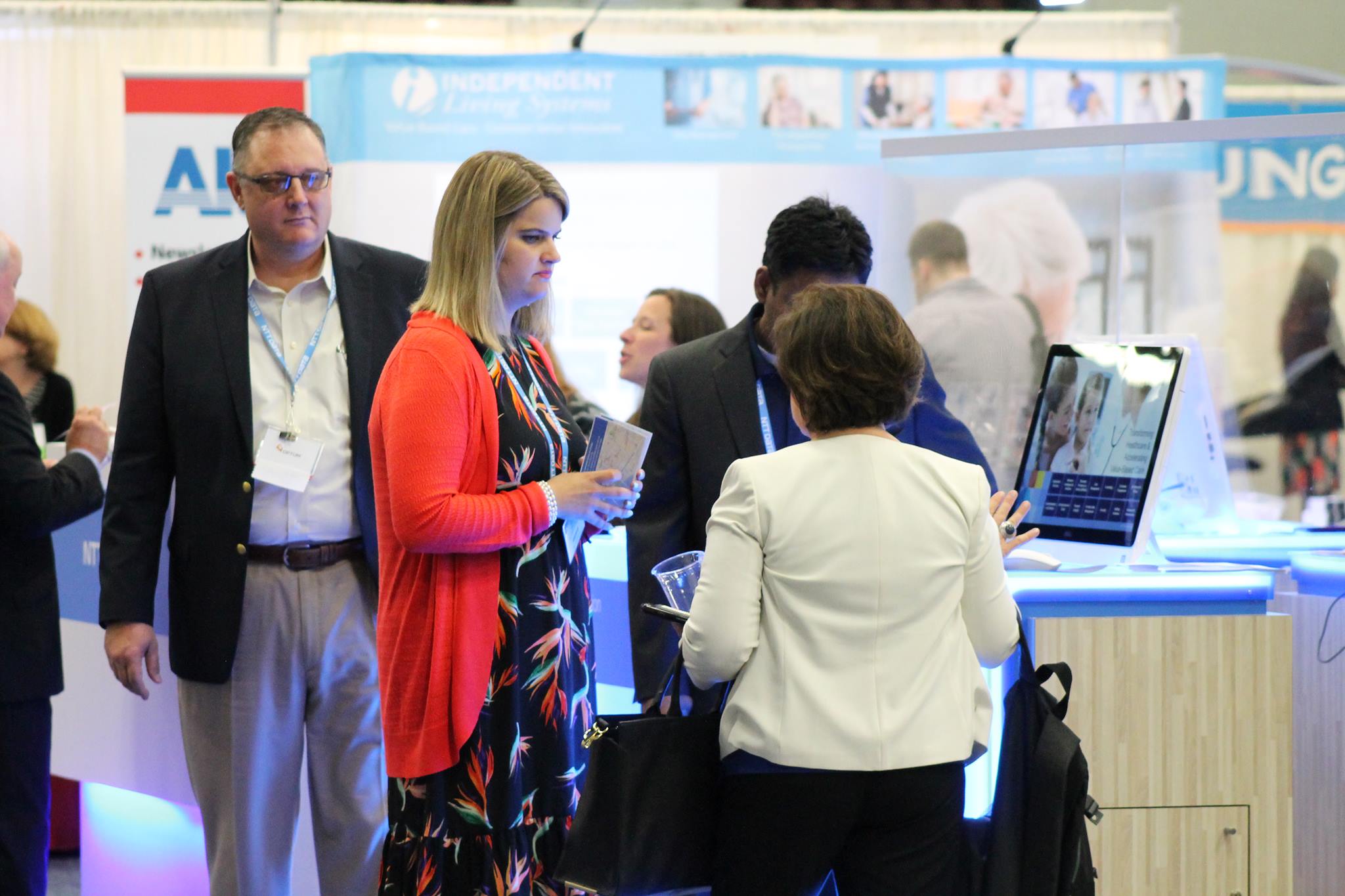
For instance, Colorado is developing rules to ensure AI systems in insurance do not discriminate against protected classes. Other states like California, Georgia, Illinois, New York, Pennsylvania, and Oklahoma are also considering similar legislation. Some states, including Maryland and Vermont, have issued guidance modeled after NAIC language, setting clear expectations for AI use in insurance.
However, insurers express concern over varying state regulations, which could complicate the implementation of uniform AI systems across the country.
How to Make AI a More Friendly Assistant?
Fortunately, in an analysis published in Health Affairs, there are several ways to check predictive models and business processes for bias, and health insurers should establish standard but flexible protocols for auditing their models and processes.
Representational Fairness
One effective method is representational fairness, which involves comparing the outreach and engagement rates in care management programs with the proportions of subgroups in the data.
For instance, if an eligible population comprises 40 percent White, 30 percent Black or African American, 20 percent Hispanic or Latino, and 10 percent Asian individuals, the outreach and engagement rates should reflect these proportions. This method helps identify representational bias, although it does not ensure equitable resource allocation based on true care needs.
Counterfactual Reasoning
Another approach is counterfactual reasoning, which assesses whether individuals from different subpopulations but with the same health profile receive the same predicted outcomes. For example, researchers found that prioritizing patients by risk scores from a predictive algorithm resulted in only 17 percent of eligible Black patients for a care management program.
By simulating corrections through counterfactual fairness, researchers could increase Black patient eligibility to 46 percent. This method evaluates fairness by comparing the treatment of different subpopulations under similar conditions, ensuring that biases related to race and other confounding factors are addressed.
Error Rate Balance and Error Analysis
Error rate balance and error analysis involve comparing false positive and false negative rates across subpopulations to identify bias. For example, a chi-square test can compare these rates by gender, and a statistically significant result indicates bias in the model’s predictions. Understanding the patterns of errors helps in improving the machine learning pipeline. Reviewing errors with diverse stakeholders provides context on why specific types of errors occur and their impact, guiding adjustments like upsampling or downsampling rates in training data or creating different models for subpopulations.
Algorithmovigilance
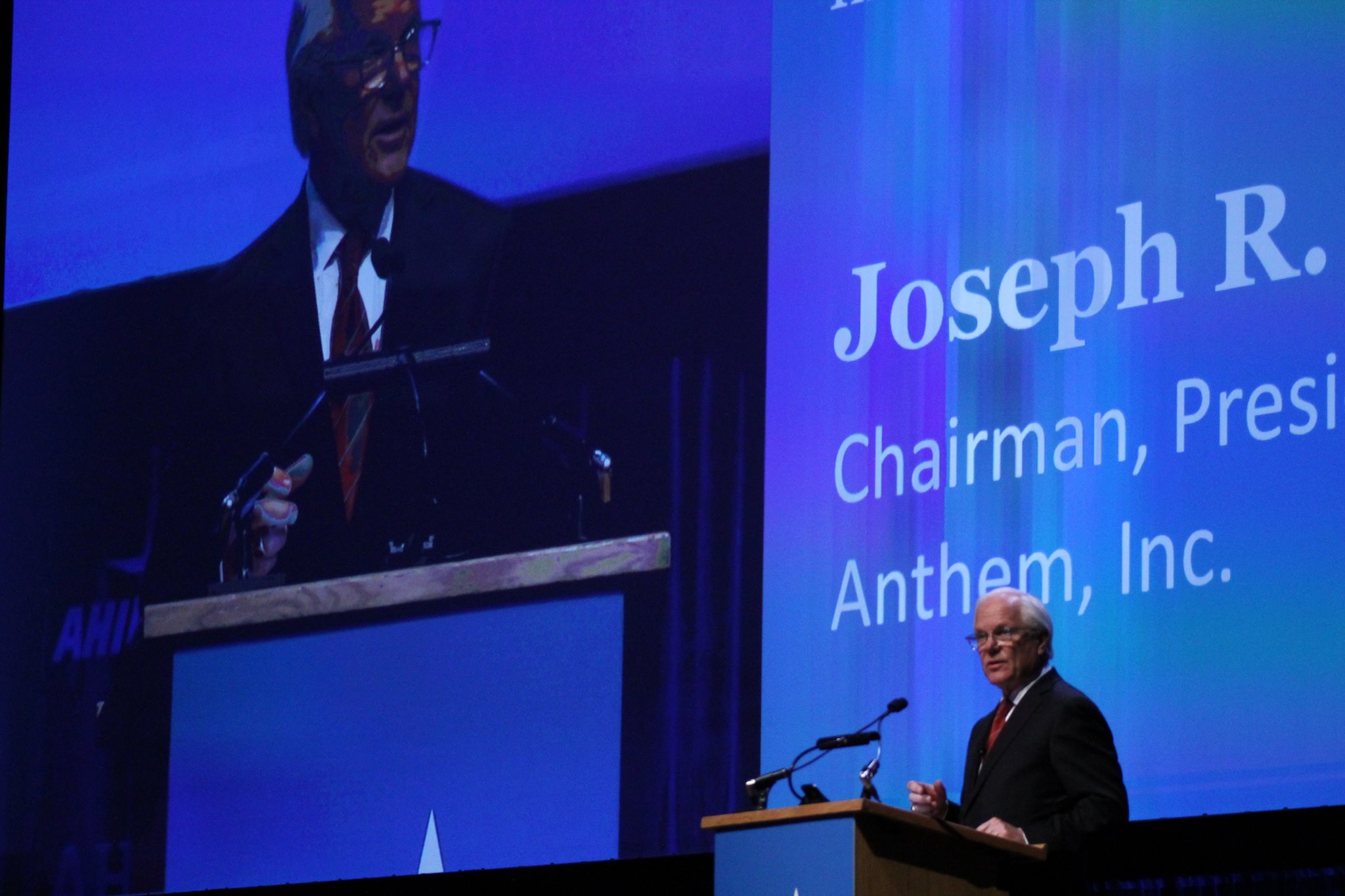
The concept of algorithmovigilance emphasizes the continuous evaluation and monitoring of algorithms for adverse effects and bias. This involves incorporating known methods for identifying and mitigating algorithmic bias into machine learning pipelines and participating in ongoing development of new methods.
Regular assessments ensure models generate insights that maximize intended outcomes, such as reducing acute hospitalizations, while maintaining fairness across subgroups. Continuous monitoring prevents “bias drift” over time and ensures interventions benefit members at the highest levels of risk and need.
Collaborative Industry Efforts
Collaborative industry efforts are essential for addressing common challenges in reducing bias. Health insurers should share best practices and develop industry-wide standards for fair machine learning.
This collaboration can lead to unified guidelines, best practices, and analytics tools to combat bias, ensuring high-quality, equitable care for members. Additionally, collecting data on race, ethnicity, and language, despite ethical and regulatory challenges, can enhance bias audits. Establishing ethical principles and standards for data collection and use, guided by entities like America’s Health Insurance Plans or the NCQA, can provide clarity and protection.
Including Diverse Voices
Lastly, including diverse voices in the development of machine learning models is crucial. Collaborative efforts between data scientists, clinical experts, and individuals with lived experiences of systemic inequities reveal blind spots and improve model fairness. Diverse teams bring valuable perspectives that help promote health equity through predictive analytics.
By incorporating these comprehensive and collaborative approaches, health insurers can effectively address and reduce bias in their predictive models and business processes, ultimately leading to better outcomes and lower costs for their members.